
Data Collection
IMDb Dataset
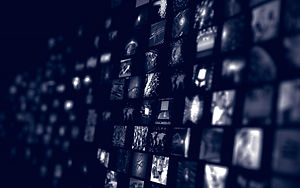
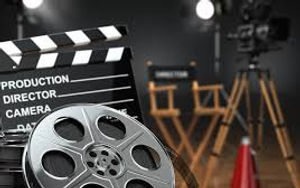
IMDb is “the world’s most popular and authoritative source for movie... content”. For the IMDb dataset, we use the advanced title search provided by the IMDb website and set up our filters as follows: “Title Type: Feature Film”,”User Rating: 7.0 to 10.0”,”Number of Votes: minimum 20000”,”Title Data: Soundtracks”. For the “Display Options”, we choose “Detailed” mode, show “250 per page” and sort the results by “Popularity Ascending”.
From the search results, we scraped titles, years, runtimes, genres, ratings, votes and the grosses for all 2122 movies, which consist of our first part of dataset.
Then we intended to use movie titles to query the corresponding soundtracks and their music attributes using the powerful Spotify API. Yet, it turned out to be troublesome. While Spotify API could accurately return the soundtrack(s) for most movies, for some of them, it failed. We detected several characteristics of failed movie names by trial and error and decided to pre-clean our movie titles to facilitate our query in Spotify.
The cleaning procedures are listed in Table 1: Undesired Patterns in Movie Titles and Clean Procedures. After cleaning, all the punctuations and special characters were formatted with space or normal letters in English. This method improved our query results. The total number of failed movie names decreases almost one thirds, from 290 to 202; the total number of collected tracks increases 4.8%, from 25098 to 26292,.
Spotify Dataset



Spotify is a digital music service that gives its user access to millions of songs. We use movies titles collected in IMDb datasets and Spotify API to scrape album data using Spotify search.
In getTracklist.py: we used the movie title in cleanMVData.csv as a searching criteria to find the corresponding soundtrack albums. The search will return multiple results in a json file. We used the topmost related result and collected the tracklists and the corresponding track id of each track in the album. We combined the track name and id with the movie information collected in the last step to create an aggregate data frame and saved it as tracklist.csv.
In getFeaturelist.py: we used the track ids in tracklist.csv to scrape feature data of each soundtrack. Our collected information includes acousticness index, danceability index, duration of the soundtrack, energy level, instrumentalness index, key signature, liveness index, loudness measure, mode, speechiness index, tempo, time signature, valence level, and popularity. Then we saved the data in feature_list.csv file.
Finally, we integrated all the data sets into the full_dataset.csv.